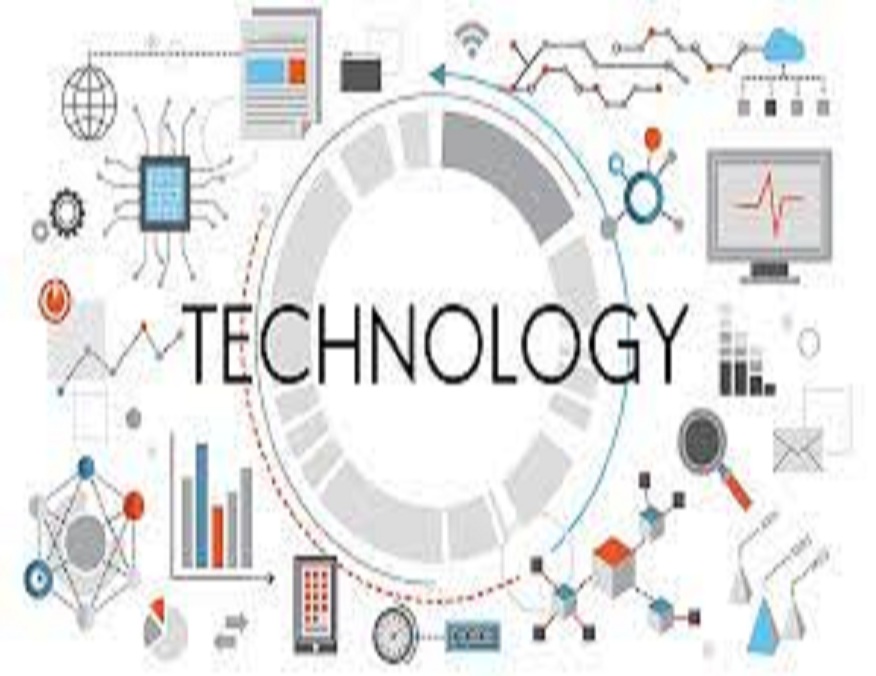
Artificial intelligence is experiencing considerable expansion and the number of technologies based on it are increasing day after day, interfering in all areas, both industrial (security, health, banking-insurance) and daily life. Endowed with a common language, these technologies inter-involve, call and respond to each other, causing on the one hand, the transformation of the sectors they penetrate, and favoring, on the other hand, the emergence of ecosystems1they govern.
Consequently, the question of the technological independence of the European Union is acutely doubled by that of its political, social and organizational independence, and this, not only because it no longer creates the technologies it can and wants to have, but also because these technologies convey values which do not necessarily correspond to the political values on which European democratic societies are based.
Awareness of this impact as systems are deployed has fueled, in the United States and in international forums, scientific research and discussions around the concrete modalities of fairness in AI while emerging at the same time the question of an algorithmic colonialism. Our purpose in this study is to show a) the political and organizational issues underlying the choice of fairness , b) the types of solution – technological, legal, political –, all of which are incomplete, c) to outline some avenues for technological and legal reflection that would make it possible to endorse a universalist, egalitarian, non-discrimination conception, congruent with European political models .
Discrimination and the birth of “fairness” in artificial intelligence
Technologies are not part of an environment without reconfiguring it in a more or less profound way – think of the infrastructure made necessary by the car – and they are not devoid of social values and standards, which they convey through the environment they colonize. Consequently, the question of the technological independence of the European Union is acutely doubled by that of its political, social and organizational independence.
These examples are relatively well known and bear witness to the tangible effect of AI technologies on people’s lives. However, apprehended as system deficiencies, they suggest or believe that without the tragedy of error, AI systems would be neutral and without discriminatory impacts. This is to forget that the prescriptive performance of AI is precisely conditioned by the discriminations – the sorting – that it operates in the diversity of data, through its algorithms. It could be objected that European liberal democracies, due to the non-registration of data related to race or religion, factually exclude themselves from algorithmic discrimination. But that would be ignoring the fact that the simple fact that the algorithm is designed to perform this type of sorting biases it axiologically.
The path of technological solutionism
The first path taken consists of internalizing the ethical problem of fair treatment and non-discrimination within the model. Mechanisms for automating the concept of fairness are developing and enriching the production chain for AI models. We thus add new means of control that most Cloud providers offer in addition to the provision of computing capacities: by identifying biases, setting up alerts to prevent algorithmic discrimination and correction of affected models or datasets.
Researchers are creating a robust theoretical framework to uncover and then address bias issues in datasets and AI models. Several metrics or statistical scores, often incompatible with each other, are thus developed to encode in mathematical formalism the different incarnations of these concepts from political philosophy. Let us illustrate this incompatibility with an example: in the United States, AI algorithms are sometimes responsible for determining the risk of recidivism of inmates. Several fairness tests of the algorithm can be considered but these metrics cannot be satisfied simultaneously:
These measures are divided into two groups according to the following division:
or the individual prevails over the group: with this Aristotelian conception, two individuals with similar characteristics must be treated by the algorithm in the same way.
or the group prevails over the individual and is perceived as a whole. In this second case, the algorithm must deal fairly with the minority group (also called the protected group according to the word-for-word translation from English) and the majority group.
These two views are irreconcilable in the sense that neither necessarily implies the other. Moreover, choosing a statistical measure according to one or other of the paradigms is a subjective choice and national legislation on discrimination in Europe is fundamentally contextual and does not specify the statistical test and the significance thresholds to be used depending on the case. of figure. The objective of this legal indeterminacy is to leave ample room for the judge’s discretion, so that case law can best support and frame the developments in artificial intelligence systems.
In the same way, what from the technical point of view appears as a “contextual vagueness” also translates a fundamental position of ethics: to be operative on a system, to remain free from it, it must preserve an exteriority which allows it to criticize its variable limits depending on the context. Internalized and automated, ethics would be no more than one constraint among others, an element of the system with diminished efficiency. Internalization would also favor the ethical models that best lend themselves to computation, in this case, utilitarianism, through the maximization of maximum utility for a maximum number of individuals.